FedGNN (KDD’ 21)
- FedGNN: Federated Graph Neural Network for Privacy-Preserving Recommendation
- Introduction
- Centraized Learning & Decentralized Learning
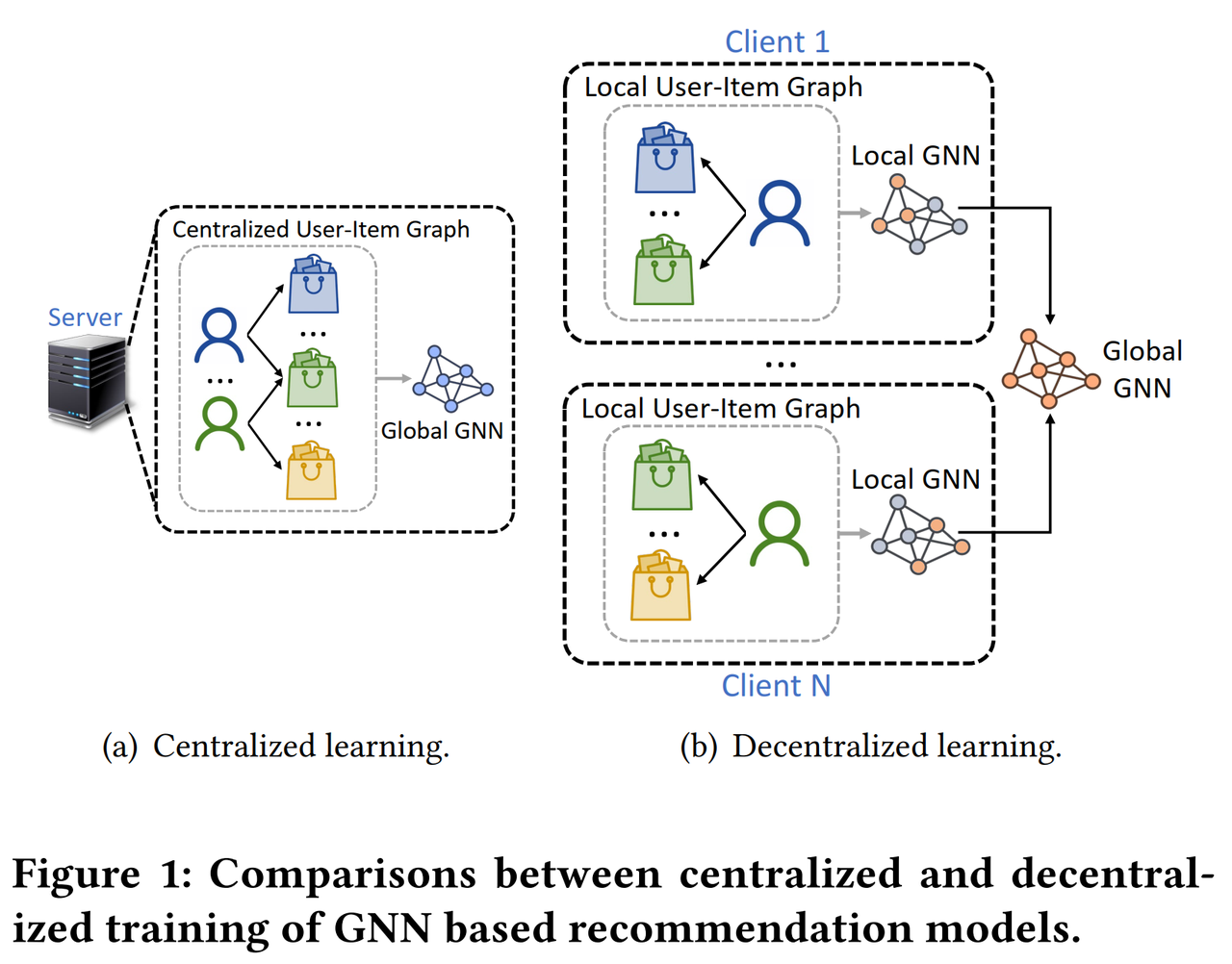
- Challenges:
- For most users, the volume of interaction data on their devices is too small to locally train accurate GNN models.
- The local GNN model trained on local user data may convey private information, and it is challenging to protect user privacy when synthesizing the global GNN model from the local ones.
- The local user data only contains first-order user-item interactions, and users’ interaction items cannot be directly exchanged due to privacy restrictions.
- Methodology
- Problem Formulation
- Users: $\mathcal{U} = {u_i, u_2, \dots, u_P}$
- Items: $\mathcal{T} = {t_i, t_2, \dots, t_Q}$
- Rating matrix: $Y \in \mathtt{R}^{P\timesQ}$
- bipartite useritem graph: $\mathcal{G}$
- $u_i$ has interactions with $K$ items: $[t_{i,1},t_{i,2},\dots,t_{i,K}]$; the ratings that given to these items: $[y_{i,1},y_{i,2},\dots,y_{i,K}]$
- FedGNN Framework
- $u_i$ -> Embedding -> $e_i^u$ -> GNN -> $h_i^u$
- Item nodes $[t_{i,1},t_{i,2},\dots,t_{i,K}]$ -> Embedding -> $[e^t_{i,1},e^t_{i,2},\dots,e^t_{i,K}]$ -> GNN -> $[h^t_{i,1},h^t_{i,2},\dots,h^t_{i,K}]$
- Neighboring user node $[u_{i,1},u_{i,2},\dots,u_{i,N}]$ -> Embedding -> $[e^u_{i,1},e^u_{i,2},\dots,e^u_{i,K}]$ -> GNN -> $[h^u_{i,1},h^u_{i,2},\dots,h^u_{i,K}]$
- Loss function: $\mathcal{L}i = \frac{1}{K} \Sigma{j=1}^K \vert \hat{y}{i,j} - y{i,j} \vert$
- Gradients of model: $g_i^m$
- Gradients of embeddings: $g_i^e$
- $g_i =(g_i^m, g_i^e)$
- Privacy-Preserving Model Update
- sample $M$ items that the user has not interacted with, and randomly generate their gradients $g_i^p$ using a Gaussian distribution with the same mean and co-variance values with the real item embedding gradients.
- $g_i =(g_i^m, g_i^e, g_i^p)$
- LDP: $g_i = clip(g_i,\delta) + Laplace(0,\lambda)$
- Privacy-Preserving User-Item Graph Expansion
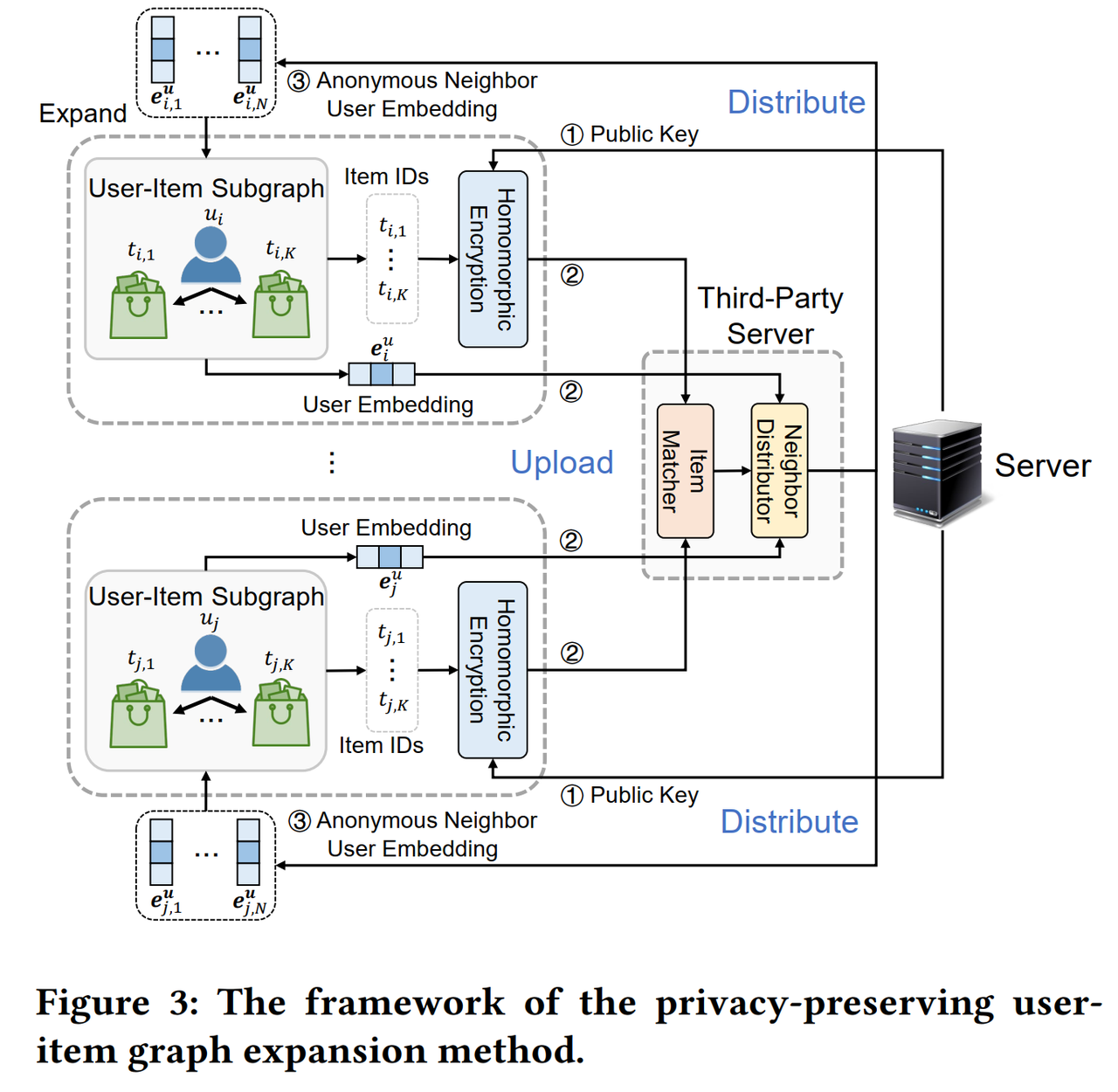
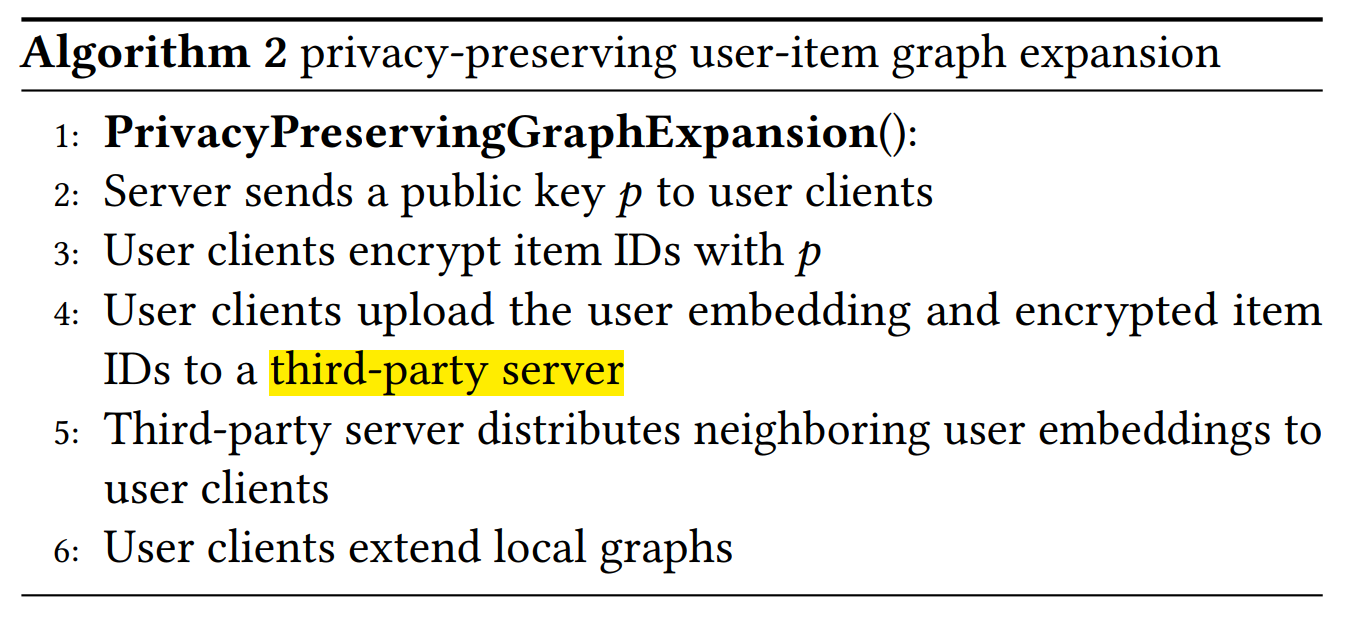
- Analysis on Privacy Protection
- If the recommendation server colludes with the third-party server by exchanging the private key and item table, the user interaction history will not be protected.
- The accuracy of model gradients will also be affected if the privacy budget is too small. Thus, we need to properly choose both hyperparameters to balance model performance and privacy protection.
Author:
Simba
License:
Copyright (c) 2019 CC-BY-NC-4.0 LICENSE
Slogan:
乳虎啸谷,百兽震惶